In healthcare, data gaps aren’t mere oversights; they pose real threats to patient safety. Imagine finding your way in a dark room without a flashlight—missing information creates dangerous blind spots that can lead to severe consequences. Recognizing and addressing these gaps is necessary for improving healthcare outcomes.
By leveraging data analytics and strong data governance, we can shine a light on these hidden risks. Enhancing our systems protects patient information and elevates the quality of care. This article will provide you with actionable strategies to identify and resolve these critical data gaps effectively.
Key Takeaways
- Identify hidden data gaps by analyzing patient demographics, care disparities, and social factors.
- Create a centralized knowledge hub and promote continuous education to improve data governance and improve communication.
- Use AI tools to analyze patient data, elevating quality performance and effectively closing care gaps.
- Develop strong incident response plans and security measures to prevent data breaches and protect sensitive health information.
Identifying Data Blind Spots in Healthcare
Identifying data blind spots in healthcare is critical for enhancing patient safety and improving healthcare outcomes. Just as a driver relies on mirrors to steer the road, healthcare professionals must scrutinize their data to reveal hidden risks. What happens when critical data points are overlooked?
Misdiagnoses and delayed treatments can jeopardize patient care.
Common blind spots include implicit bias and confirmation bias, which can skew healthcare analytics. For example, if a hospital’s data primarily reflects certain demographics, it may neglect the needs of underrepresented groups. This is like baking a cake without all the ingredients—something critical is missing!
To combat these issues, we can do strategies such as data governance, ensuring thorough data collection and analysis. Regular audits of electronic health records (EHRs) are necessary for maintaining data quality and enhancing interoperability. Moreover, utilizing machine learning and predictive analytics can uncover hidden patterns, remarkably boosting clinical decision support.
By identifying and addressing data blind spots, we create a safer and more effective healthcare environment.
Assessing the Impact of Data Gaps on Patient Safety
In healthcare, data gaps are like missing puzzle pieces, obscuring the full picture of patient safety. What happens when critical information goes unrecorded?
Decisions made on incomplete data can result in severe adverse events for patients.
This is where healthcare analytics comes into play. By analyzing vast amounts of healthcare data, we can identify patterns that improve clinical decision support. But, when data is missing, our analyses falter. This is why data governance is critical—it ensures that our electronic health records (EHRs) are both accurate and complete, promoting interoperability across different systems.
Furthermore, consider the ripple effect of data gaps. Just as a single missing link can weaken a chain, absent data can compromise the entire healthcare system. Ensuring strong data collection and management not only protects patients but also empowers healthcare providers to make well-informed choices. How can we afford to overlook this critical aspect of care?
In the end, addressing data gaps is not just a technical challenge; it’s a moral imperative. By prioritizing data integrity, we can remarkably improve patient safety and improve in general health outcomes. Are we ready to fill in the gaps and safeguard our patients?
Implementing Effective Data Governance Strategies
Implementing effective data governance strategies is critical for eliminating data blind spots in healthcare. Imagine a ship finding your way through dense fog; without clear visibility, it risks disaster. Healthcare organizations face similar dangers when data is unclear or inconsistent. How can we make sure our data serves its critical purpose?
Begin by establishing a strong data governance framework. This framework defines roles and responsibilities, ensuring everyone knows their part in maintaining data quality and patient safety. By utilizing automated tools and machine learning algorithms, we can quickly spot and correct errors, much like a coach reviewing game footage to increase team performance.
Enhancing data integration and promoting interoperability among systems allow seamless data sharing. This transparency bolsters healthcare compliance and improves clinical decision support. By prioritizing data privacy and information security, we effectively reduce risks tied to data management.
A strong commitment to data stewardship leads to improved healthcare outcomes, ensuring we don’t just manage data but harness it wisely to improve patient care.
Utilizing Data Analytics for Gap Detection and Resolution
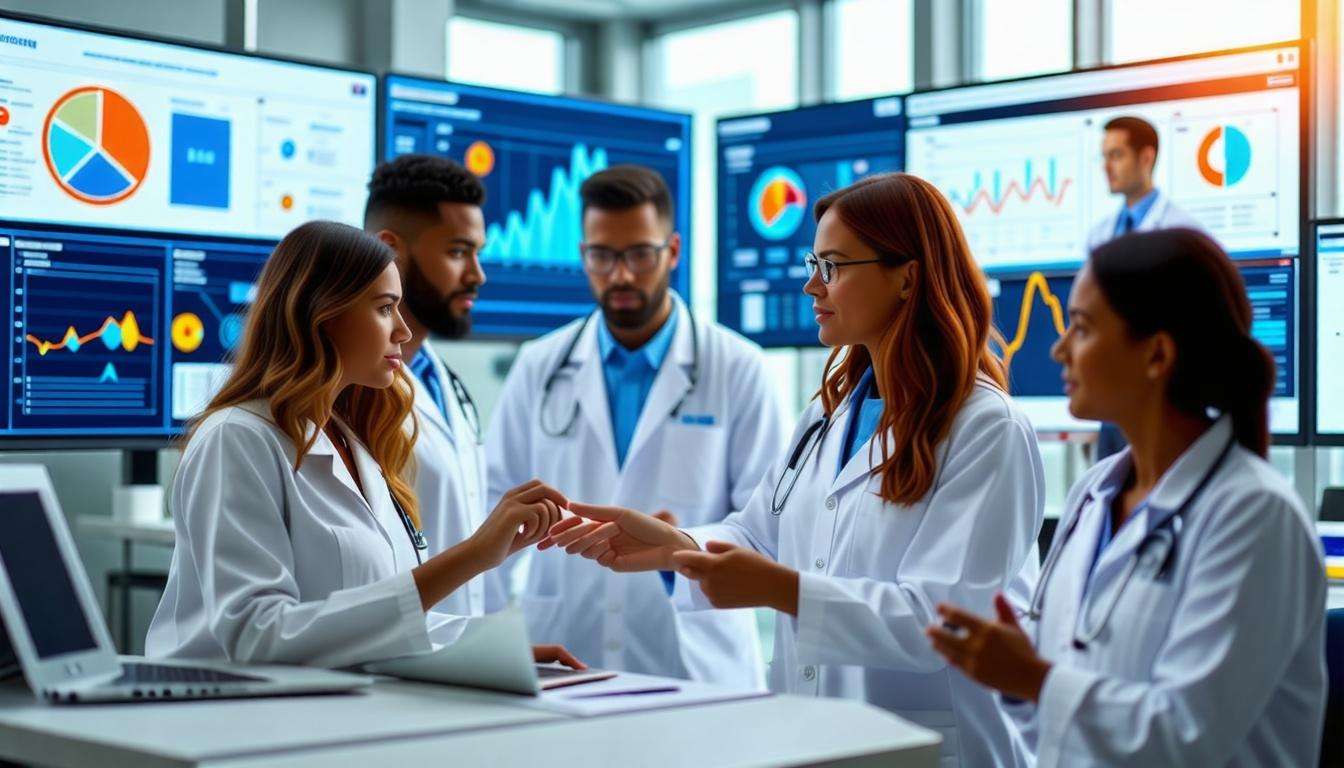
In the area of healthcare analytics, identifying and addressing data blind spots is critical for enhancing patient safety and improving healthcare outcomes. Think of these blind spots as hidden potholes on a road; without awareness, we risk veering off course. So, how can we use data analytics to uncover these gaps?
Initial, implementing strong data governance strategies is necessary for ensuring the quality and integrity of our electronic health records. Regular audits and assessments help us detect inconsistencies. By utilizing predictive analytics, we can foresee potential risks, empowering us to make well-informed choices that improve clinical decision support.
Integrating machine learning and artificial intelligence into our systems enables us to analyze vast data sets efficiently. This not only boosts operational efficiency but also refines our gap detection and resolution strategies. For instance, data visualization techniques can reveal patterns that might otherwise go unnoticed.
By accepting a data-driven decision-making approach, we can effectively close the gaps in healthcare data, paving the way for a safer and more effective healthcare system for everyone.
Enhancing Interoperability and Data Integration Practices
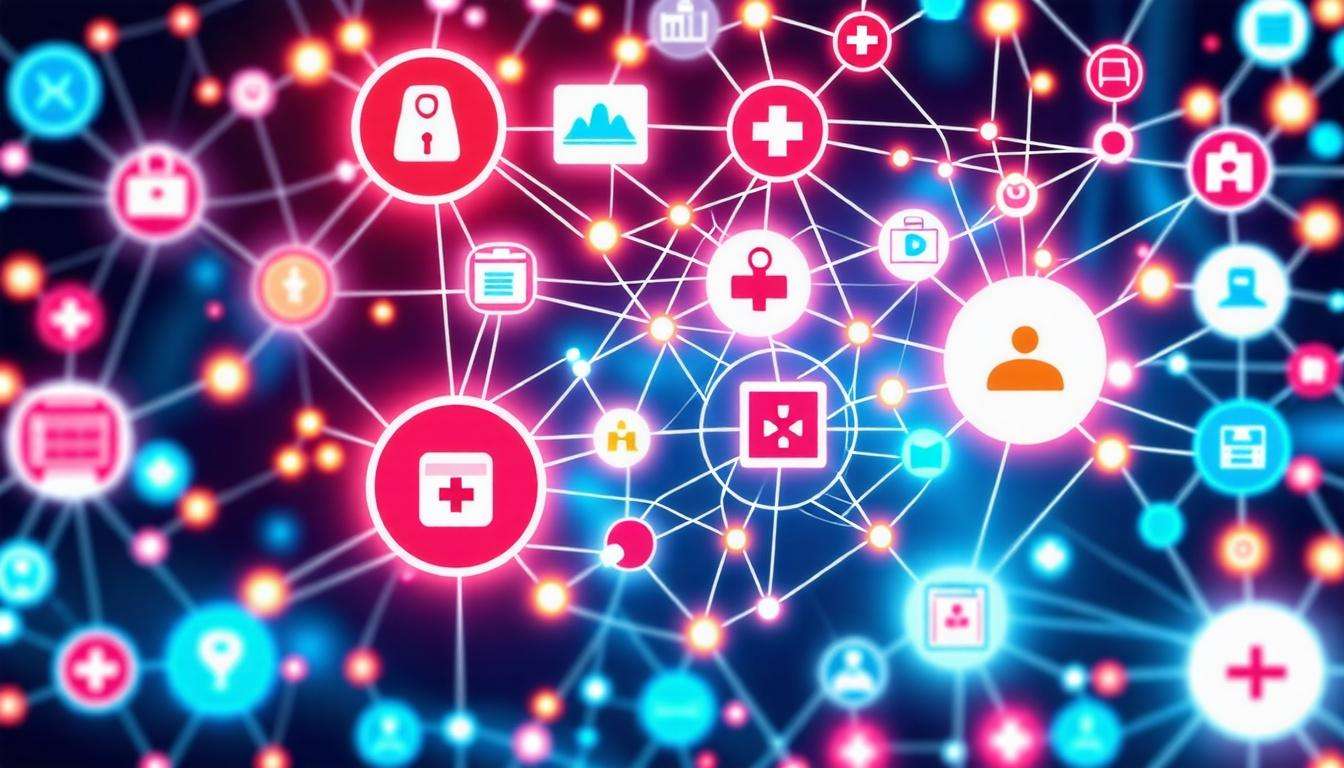
Enhancing interoperability and data integration in healthcare is akin to conducting a symphony. Each instrument—representing diverse systems and data sources—must harmonize to optimize patient outcomes. Why is this cooperation critical?
Data gaps can jeopardize patient safety and skew healthcare outcomes.
To tackle these issues, strong data governance and effective health information exchange are necessary. Consider these key strategies:
- Standardization: Implementing interoperability standards facilitates seamless communication between systems.
- API Integration: Use API integration for real-time data sharing, enhancing clinical decision support.
- Data Quality: Regular audits and updates are critical for improving data quality and reducing inaccuracies.
Moreover, technologies like artificial intelligence and machine learning can predict risks and streamline workflows, minimizing human error. Just as a garden flourishes with care, our healthcare data systems thrive through meticulous data analytics and strategic integration practices.
Strategy | Benefit |
---|---|
Standardization | Enhances communication |
API Integration | Improves decision support |
Data Quality Audits | Lowers inaccuracies |
Leveraging Artificial Intelligence and Machine Learning for Predictive Analytics
Leveraging Artificial Intelligence (AI) and Machine Learning (ML) for Predictive Analytics is akin to having a crystal ball in healthcare. Imagine predicting patient needs before they arise! This foresight is critical for addressing data blind spots that jeopardize patient safety.
Healthcare data is vast, yet much of it remains untapped. Ensuring data quality and interoperability is critical for revealing necessary insights. With AI algorithms, we can steer through electronic health records, uncovering trends that might otherwise stay hidden—like finding gems in a sea of information.
Predictive modeling enhances clinical decision support, equipping healthcare professionals to make informed choices. Regular audits and validation of AI systems are necessary to reduce biases, ensuring fair and accurate outcomes. Can you picture the transformative impact of a seamlessly integrated healthcare system on population health management? It’s nothing short of revolutionary!
AI & ML Benefits | Impact on Healthcare |
---|---|
Enhanced Data Analysis | Identifies trends in patient care |
Improved Patient Outcomes | Reduces risk through timely interventions |
By utilizing the strength of AI and ML, we can transform healthcare analytics, paving the way for superior risk management and data governance. This strategy protects data privacy and elevates in general healthcare outcomes.
Summing up
Closing dangerous data gaps in healthcare is necessary for ensuring patient safety and delivering quality care. By utilizing the strength of artificial intelligence and machine learning, we can identify these gaps and remarkably improve data quality. Regular audits and validation of AI systems are not just significant; they are critical for ensuring fairness and accuracy in healthcare analytics. Strengthening our data governance and integration unlocks the potential for better health outcomes and regulatory compliance. Together, we can build a safer, more efficient healthcare system that benefits everyone.
Frequently Asked Questions
What can the hospital do to reduce the risk of blind spots in the ward?
Hospitals can reduce blind spots by implementing regular data audits, enhancing AI validation, and fostering multidisciplinary collaboration.
How to identify gaps in healthcare?
Conduct regular audits, use AI for data analysis, and engage multidisciplinary teams to uncover and address data gaps effectively.
How can technology help in identifying healthcare data gaps?
Technology, like AI and ML, can analyze vast datasets, uncover biases, and highlight gaps, ensuring better healthcare outcomes.
What are common causes of data gaps in healthcare?
Common causes of data gaps in healthcare include inconsistent data entry, lack of interoperability, outdated systems, and human errors.